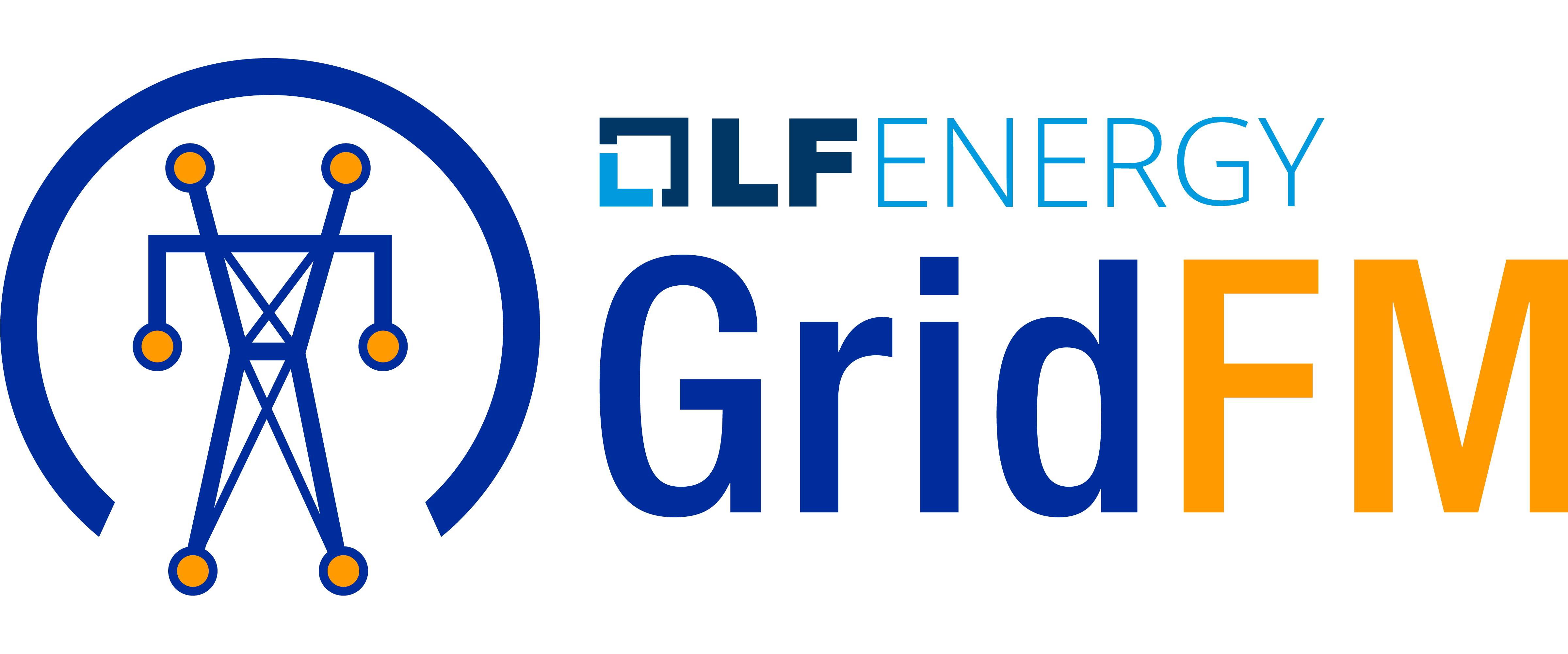
GridFM is an open source framework to enable the emergence of foundation models for power grids.
Foundation models (FMs) are large artificial intelligence models pre-trained on large data sets and adapted to a broad set of applications. Powerful FMs for language and weather have proved that such models can be developed for complex systems. FMs for the electric power grid (GridFMs) have similarly been conceptualized to be trained on grid data.
GridFMs have the potential to cope with the increasing complexity and uncertainties stemming from the energy transition by providing a significant speed-up in computation. A key benefit of FMs is their ability for stakeholders to fine-tune a pre-trained model for specific applications, using their own proprietary data in a scalable and economical way.
This makes GridFM an ideal technology platform to collaborate across the energy sector, academia and AI specialists to harness emerging AI capabilities for a variety of applications.
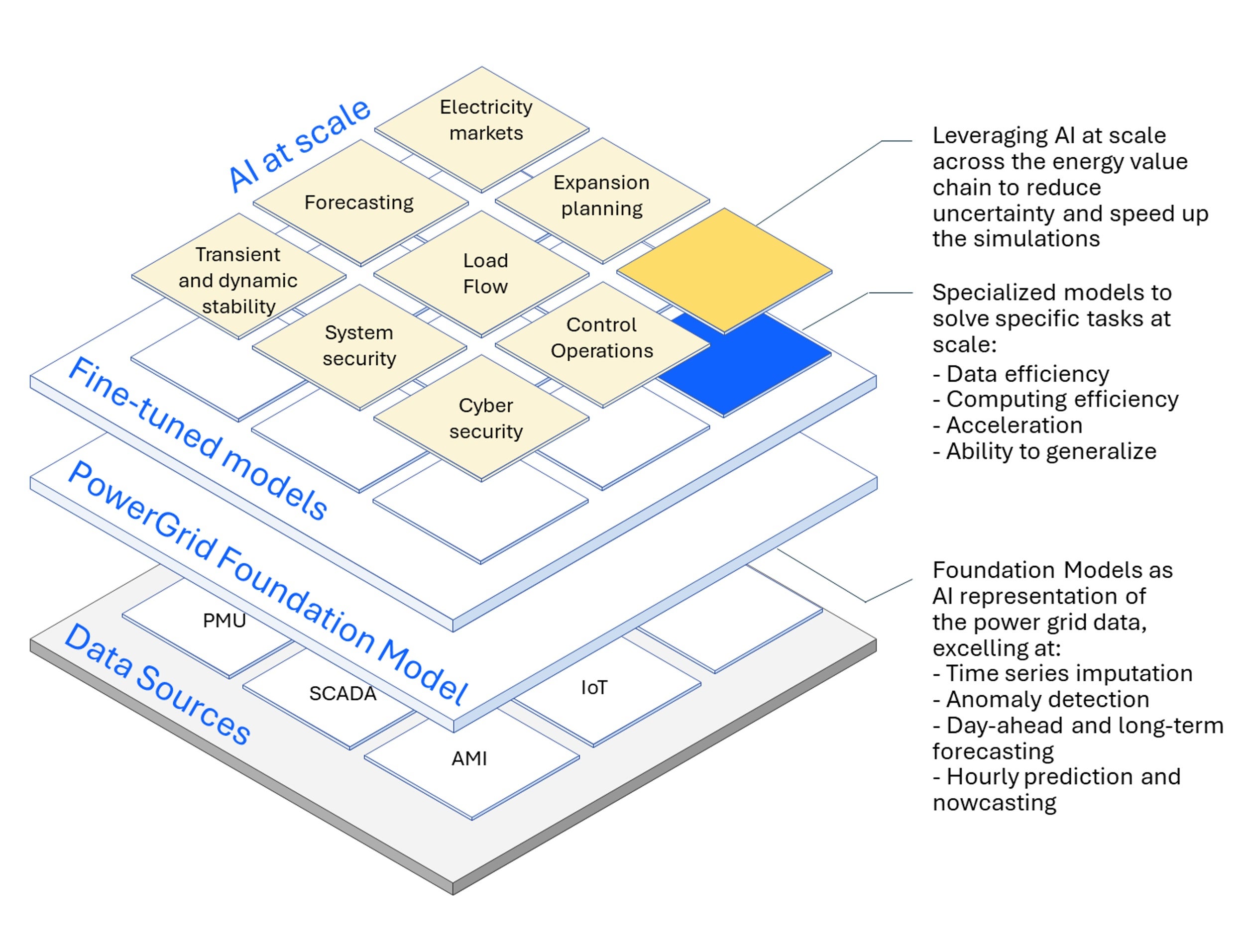
Fig 1. The GridFM stack
Initially, the project will focus on establishing a large collection of solved AC power flow for different grid topologies, parameters, and different load conditions.
In the second phase, suitable architectures will be evaluated by pre-training performance analysis, and by adjusting models, training strategies, and loss functions. GridFM-v0 will be pre-trained to reconstruct masked power flow data as illustrated below.
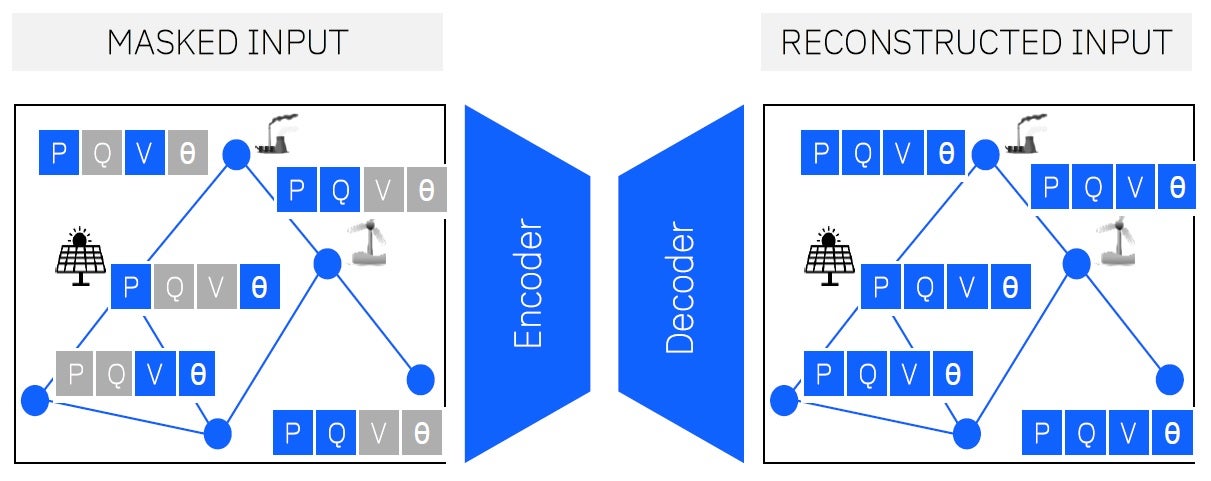
Fig 2. Masked power flow data
This first model will be tested and fine-tuned with data located behind utility firewall for downstream applications closely related to power flow like complementing numerical power flow solvers, contingency analysis and optimal power flow.
In the long-term, we envision expanding and addressing more grid applications by including more diverse data modalities to pre-train a single GridFM or by combining different GridFMs.

Fig 3. GridFM Roadmap
Contributors
- Hydro-Québec
- IBM